Hey there, fellow tech enthusiasts! Today, we’re diving into some exciting developments in the world of artificial intelligence, specifically how AI is learning to think more like us humans. Ever wonder what it takes for a machine to make decisions as we do? Well, buckle up, because researchers from Georgia Tech have just made some groundbreaking strides in this area with their new neural network, RTNet.
Imagine this: on any given day, a typical person makes around 35,000 decisions. From choosing your breakfast to determining whether it’s safe to cross the street, decision-making is an intricate dance of evaluating options, weighing past experiences, and gauging our confidence in our choices. Now, that might sound overwhelming, but for most of us, it’s second nature. But machines? They’ve been lagging behind, making the same decisions time and again without any nuance.
Breaking Down the Human Decision-Making Process
While we humans rely on a mix of intuition, experience, and even a touch of uncertainty in our decision-making, traditional neural networks have often lacked this sophistication. They usually give you the same answer under the same conditions—boring, right? That’s where the genius of Georgia Tech’s research comes in. Led by Associate Professor Dobromir Rahnev, the team is on a mission to train neural networks to emulate our decision-making flair.
In a recent paper published in Nature Human Behaviour, the researchers unveiled their novel neural network designed to not just replicate human decision-making but to understand and express confidence—think of it as an AI that’s not afraid to admit when it doesn’t know something!
The Key Differences: Confidence and Variability
Farshad Rafiei, who earned his Ph.D. in psychology at Georgia Tech, emphasized a crucial distinction: “Neural networks make a decision without telling you whether or not they are confident about their decision.” This lack of transparency can sometimes lead to what’s known as hallucination in large language models (LLMs). For instance, if you ask an LLM a question outside its knowledge scope, it might create a plausible-sounding answer, while a human would probably just say, “I don’t know.”
By integrating human-like decision-making processes into RTNet, the goal is to avoid such missteps and improve AI’s accuracy in interpreting and responding to questions.
Training the Neural Network
So, how did these researchers design and test RTNet? They started by training it on the classic MNIST dataset, which is a collection of handwriting samples containing digits. The unique twist? They layered noise onto the digits to throw things off a bit, making it more challenging to decipher. They then compared RTNet’s performance with other models (CNet, BLNet, and MSDNet) to see who could decipher the digits best.
RTNet is built on two significant principles: it employs a Bayesian neural network (BNN) that utilizes probability for decision-making while also implementing an evidence accumulation process that tracks information for each choice. This allows the network to exhibit variability in its responses, much like how humans might hedge their bets based on previous experiences or new evidence. Once it feels confident enough, RTNet makes its final call.
They even factored in decision-making speed, analyzing how the model adhered to the “speed-accuracy trade-off” that dictates we humans can get a bit sloppy when rushed.
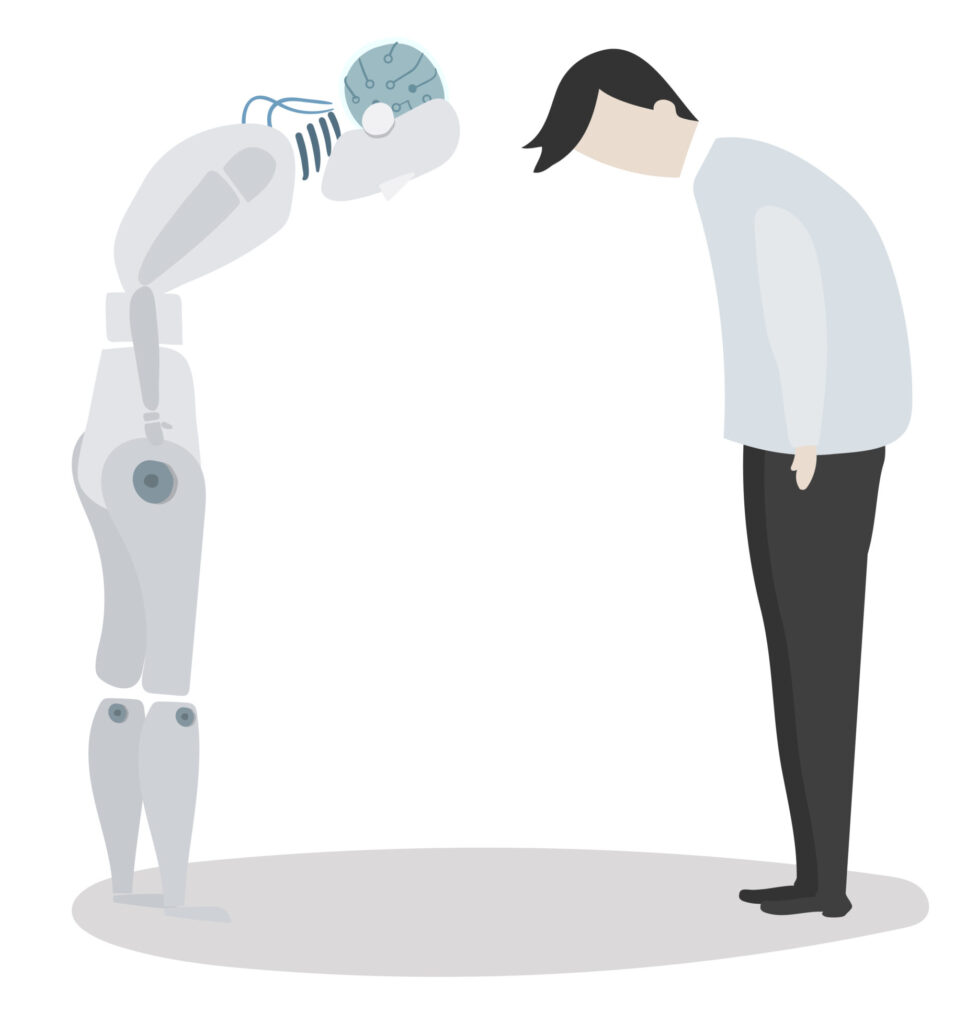
The Results Are In!
After putting RTNet through its paces, the researchers gathered insights from 60 Georgia Tech students who analyzed the same dataset. Interestingly, the results showed that their neural network’s accuracy, response time, and confidence levels were surprisingly similar to those of the students. Talk about a win-win!
As Rafiei put it, “Generally speaking, we don’t have enough human data in existing computer science literature, so we don’t know how people will behave when they are exposed to these images.” This gap is crucial, as understanding human behavior can significantly enhance AI models to replicate our decision-making processes more accurately.
AI vs. Human: The Forever Debate
So, is AI better than humans? Can it beat us at our own game? While AI continues to evolve and showcases significant potential in areas like digit recognition and decision-making, the human touch—our ability to adapt, feel, and express uncertainty—is still a substantial advantage in many contexts. Yet, with innovations like RTNet, the line between our cognitive processes and artificial intelligence is becoming increasingly blurred.
Conclusion
The team’s model didn’t just outshine other deterministic models; it also showed better accuracy in high-speed situations because it mimics human psychology. RTNet, for example, naturally boosts its confidence with correct decisions, and that wasn’t even a specific training goal. “If we shape our models to act like the human brain, they’ll reflect that behavior without extra fine-tuning,” Rafiei explained. The researchers plan to expand their dataset to explore the model’s capabilities further and apply this Brain-like Neural Network (BNN) approach to other models to enhance their decision-making. Ultimately, the goal is to see if AI can beat humans, potentially easing the mental load of the countless decisions we face every day. In summary, as AI becomes more human-like, it could revolutionize how we handle daily choices.